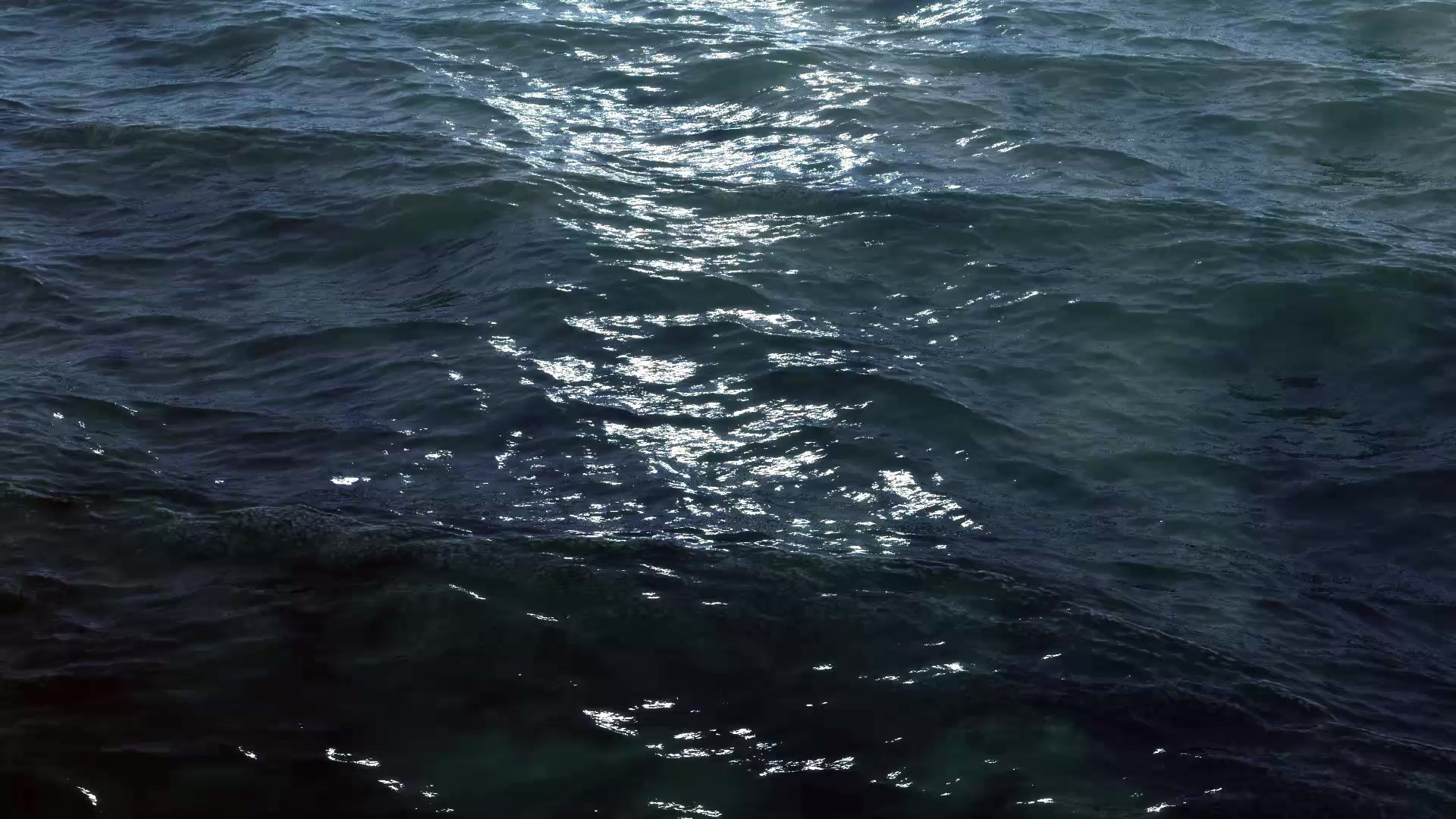
BMEG
A CONFIDENT DIAGNOSIS
OUR TECHNOLOGY
01 / FAST
Our eye-tracking and data processing software can acquire, analyze, and potentially diagnose a subject based on their eye movements in under 2 hours.
02 / UNBIASED
Our method of diagnosis is purely data analysis-based, which minimizes human bias in diagnosing subjects.
03 / EASY
Eye-tracking experiments are very easy to conduct - all it takes for the subject is looking at images!
1) The SVM classifier became repurposed to generate saliency weight per individual as opposed to data sets
Milestones
4) Parameter Optimization
​
-
positive points per image: points taken from high-density areas
-
negative points per image: from low-density areas​​
-
3:1 negative to positive pts resulted in 88% accuracy
-
Kernel Standard Deviation: ideal size of 15 for 81% accuracy
-
Kernel Size: ideal size of 30x30 for 81% accuracy
​
5) Combined Parameter Tests: Size was tested in combination with standard deviation, negative pts per image testing was combined with positive pts per image
​
6) Independent Stage Tests: proved participants change gaze over time: a baseline for cross predictions
​
7) Cross Prediction Stage Tests: using one segment of time data to predict the next​
-
accuracy must be improved upon as high variance in statistical significance persisted
-
parameters must change overtime
​
2) Overlaying Image Code: code to plot eye-tracking data onto the original image
​
3) Gaussian Blobs with Conv2: scale-space representation of the signal displayed on the original image
​
​
OVERVIEW

OUR PURPOSE
No simple test, such as a blood test, exists for Autism. The current diagnosis is by the patient’s history and behavior. An early diagnosis leads to a better prognosis. Unfortunately, children are not receiving a diagnosis until adolescence or later, lacking an education catered to their needs. Our objective was to design a machine learning-based diagnostic tool functioning on input data from an eye-tracker. Eye-tracking data is a window into the mind

OUR VISION
Past research has shown those with ASD do not exhibit as high of an interest in semantic-level features as do those without ASD. Thus, a distinguishing factor exists and became of high interest to the team's vision. If somehow the patient's gaze could be tracked over time, and this data could be converted to numerical data for analysis, a diagnosis could be made probable by machine-learning techniques.

OUR TECHNOLOGY
Our data collection began as the patients viewed 700 images. The eye-tracker recorded the eye's, and thus the mind's, interest in stimuli. Data was collected from 20 patients with ASD and 19 controls. Visual gaze activity was overlaid onto each image with respect to each eye. The overlaid images were convoluted, outputting fixation density maps. A fixation density map is a Gaussian “blob” representation of stimuli. These maps were input for an SVM classifier. The classifier trains and tests to output saliency weights per image feature. The classifier was then made to predict saliency weights according to time segmentation. Accuracy during these trials was low, yet upon renovations, the end-result remains promising.
Meet the Team
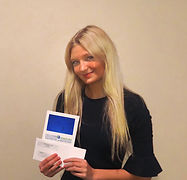.jpg)
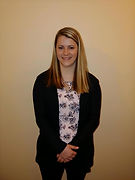
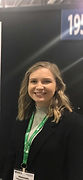
Ashley Gall
BS Biomedical Engineering
Kasey Freshwater
BS Biomedical Engineering
BA Chemistry
Hannah Cohen
BS Biomedical Engineering
Minor in Music Composition

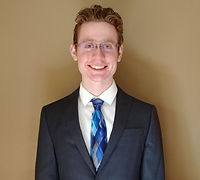
Wesley Roberts
BS Biomedical Engineering
Michael Ream
BS Biomedical Engineering
GET IN TOUCH
Benjamin M. Statler College of Engineering and Mineral Resources
Phone:
304-293-4821
Headquarters:
1306 Evansdale Dr, Morgantown, WV 26506